Deep learning helps to find new targets in soy for crop improvement
New tools, including a deep learning model, predict the functionality of proteins, even in non-model organisms
August 3, 2023
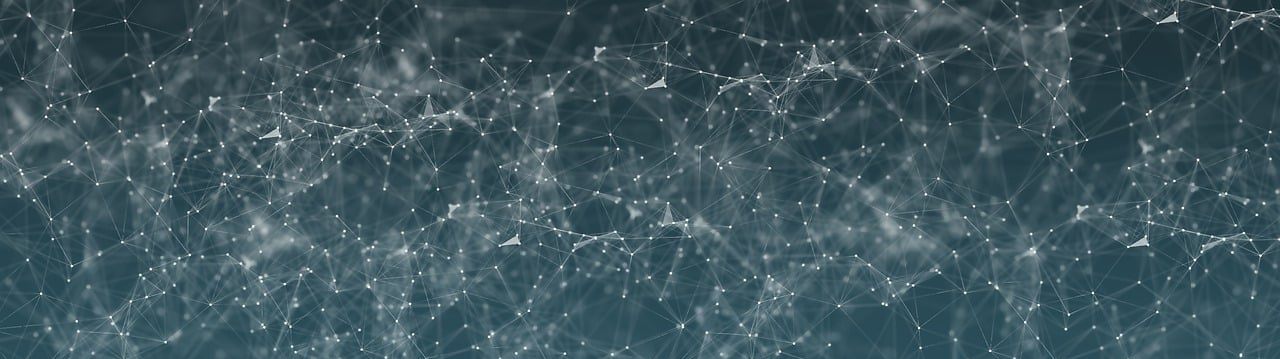
Ghent, 3 August 2023 – Crop genetic diversity is a powerful tool to adapt crops to a changing climate. However, to select the best candidates in the plant breeding process, we must know and understand the complex biochemical networks underlying plant growth and development. To achieve this, researchers from the North Carolina State University (USA), the VIB-UGent Center for Plant Systems Biology and ILVO (Belgium) have developed tools, including a deep learning model, that predict the functionality of proteins, even in non-model organisms. This represents a significant advance in systems biology and provides more reliable predictions on which locations to target in the genome. The work appears in Nature Communications.
It's complicated
Another summer, another heatwave. It's not only humans and other animals who are coping with the detrimental effects of a rapidly changing climate, the crops we rely on for food do as well. As a result, the need for crops adapted to climate change increases every year. Breeding and biotechnology could help to improve plant resilience to challenges like heat and drought. But where to start? Which genes and proteins determine these responses? The molecular basis for principles like growth and development is rarely dependent on just one protein but on complex, interconnected biochemical networks.
“These biochemical networks have been studied extensively in model organisms, but this is not always fully representative for other non-model organisms like soy, maize, and rice. The translation to more commercial, non-model organisms is not always easily made. To bridge this gap in knowledge, we use computer programs to make predictions on the functionality of certain proteins.” - Prof. Rosangela Sozzani, NCSU.
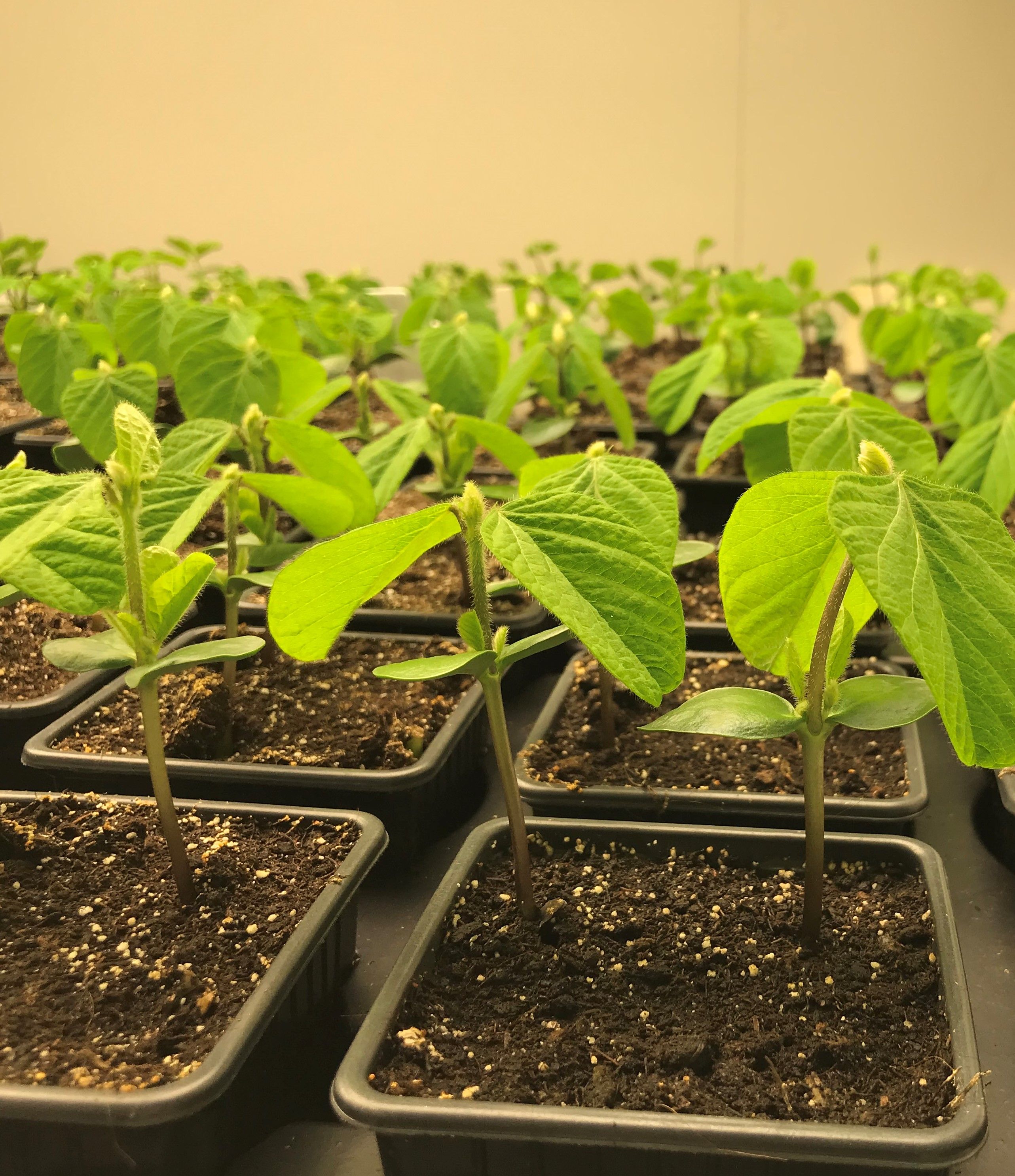
Target acquired
Researchers from North Carolina State University and United States Department of Agriculture (USDA)'s Agricultural Research Service (USA) and VIB, ILVO, and UGent (Belgium) developed a new tool to predict the functionality of a protein by using a multi-layer neural network – a form of deep learning. Based on the amino sequence of the protein, which in turn is based on a gene sequence, the webtool classifies the proteins into similar families and predicts its function. It can also identify new proteins with interesting functions. A second tool, NetPhorce then puts together the biochemical networks.
"The combination of different computational tools leads to highly confident predictions. With the help of our tool, we can identify novel functional proteins that are otherwise missed by existing methods" – Dr. Lisa Van den Broeck, NCSU
To put the tools to the test, the phosphorylation pathways in soy under cold stress were studied. Phosphorylation cascades play an essential role in reacting to environmental and cellular signals. When planting earlier in the season, as some growers prefer, or when trying to grow soy in more northern climates, cold is an important stress factor during the early growth of the plant. A potential regulatory mechanism for hot and cold stress was identified that functions as a thermostat. Additionally, two cold-specific regulators were identified. This illustrates the potential of the approach to discover new candidates for crop improvement.
"The newly annotated proteins we found were missed by previous computational models. Deep learning helped to provide a framework to classify these proteins. In addition, the biochemical network we generated provided unprecedented insight in cold signaling in soy." – Prof. Ive De Smet, VIB-UGent
“Deep learning models combined with traditional approaches provide a powerful framework for protein function annotation, signaling network inference, and understanding complex biological processes. The implications for deep learning-supported developments in biotechnology and agriculture will help us tackle the many challenges climate change presents.” - Dr. Anna Locke, USDA
The work was supported by the Foundation for Food and Agriculture Research, Benson Hill, VIB, BASF, the United Soybean Board, the North Carolina Soybean Producers Association, the National Science Foundation, and the Research Foundation – Flanders.
Publication
Van den Broeck et al. Functional annotation of proteins for signaling network inference in non-model species. Nature Communications, 2023.